Machine Learning for Diffraction Image Analysis
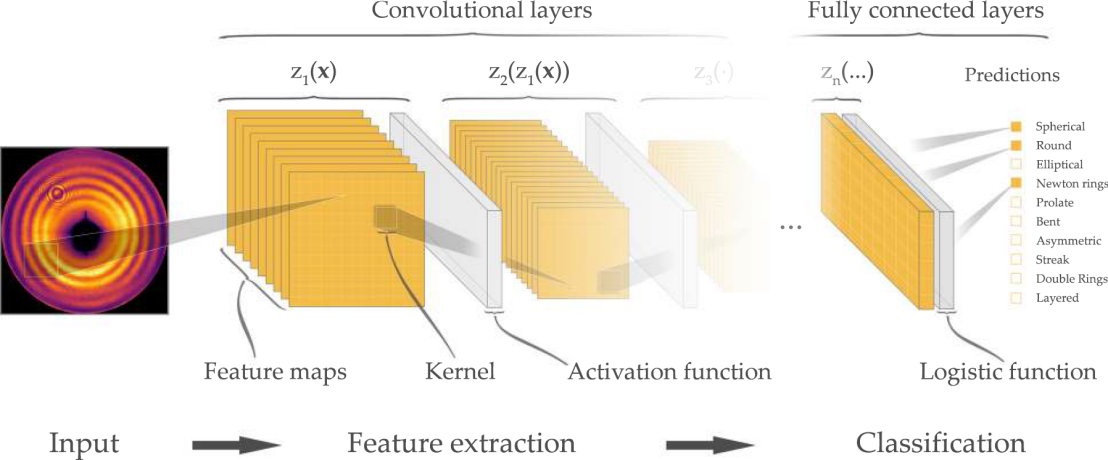
Diffractive imaging of individual nanoparticles creates huge data sets which are necessary for successful structure determination. Often up to several million diffraction patterns are recorded in a single experimental campaign. This represents a significant problem for data analysis. Usually, hand-made algorithms are developed to search for particular features within the data with the goal to filter out a certain type of events, but such approaches do not generalize well to other datasets and are very time-consuming.
We have found (external page Langbehn2018, external page Zimmermann 2019) that deep neural networks can be used to classify large amounts of diffraction data if a smaller subset of the data is labeled by a researcher and used for the training of the network. Our deep neural networks significantly outperform previous attempts for sorting and classifying complex diffraction patterns. We are currently further developing these approaches, also towards unsupervised neural networks, attempting to significantly improve post-processing and the analysis of large amounts of experimental coherent diffraction imaging data.